Original Article |
| 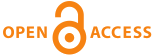 |
Applications of Artificial Intelligence and Machine Learning in Emergency Medicine Triage - A Systematic Review
Qasem Ahmed Almulihi, Abdulaziz Adel Alquraini, Fatimah Ahmed Ali Almulihi, Abdullah Abdulaziz Alzahid, Saleh Saeed Al Jathnan Al Qahtani, Mohamed Almulhim, Saeed Hussain Saeed Alqhtani, Faisal Mohammed Nafea Alnafea, Saad Ali Saad Mushni, Nasser Abdullah Alaqil, Mohammad Ibrahim Faya Assiri, Nisreen H. Maghraby. Abstract | | | | Background: Overcrowding in Emergency departments adversely impacts efficiency, patient outcomes, and resource allocation. Accurate triage systems are essential for prioritizing care and optimizing resources. While traditional methods provide a foundation, they often lack precision in addressing modern healthcare complexities. Artificial intelligence (AI) and machine learning (ML) offer advanced capabilities to enhance triage accuracy, improve patient prioritization, and support clinical decision-making, addressing limitations of conventional approaches and paving the way for adaptive triage solutions. Objective: This systematic review aims to assess the use of artificial intelligence (AI) and machine learning (ML) in determining the outcomes of patients presenting in Emergency department (ED) triage. Methods: A systematic search was conducted on April 21, 2023, using electronic databases including PubMed/Medline, Cochrane Library, Ovid, and Google Scholar, without year restrictions. The main outcome of this review was to assess the use of AI and ML in the ED Triage. Articles that used different models of AI and ML to predict various outcomes of patients in the ED setting were included. Results: A total of 17 studies were included in this systematic review. Fifteen studies assessed the role of machine learning methods in emergency department triage, while two studies evaluated the role of AI and machine learning in prehospital triage. The results of our systematic review favor the use of machine learning methods and artificial intelligence in emergency triage. Machine learning models were found to be superior to conventional emergency severity score methods in determining triage, diagnosis, and early management of patients. Among the machine learning methods, the boosting model was slightly more effective. Conclusion: Our study supports the notion that AI and ML are the future of Emergency departments. They aid in predicting patient outcomes and determining appropriate management strategies more efficiently, thereby enhancing decision making in the ED.
Key words: Artificial intelligence, machine learning, Emergency department
|
|
|
|