Original Article |
| 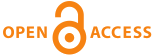 |
A Novel Instance Segmentation Algorithm Based on Improved Deep Learning algorithm for Multi-object ImagesSuhaila Farhan Abuowaida, Huah Yong Chan, Nawaf Farhan Alshdaifat, Laith Abualigah. Abstract | | | | A deep learning network is highly common and attractive in recent years because of encouraging achievements in many areas. Deep learning lies in image-based detection, and instance segmentation of an entity is a critical issue that needs further investigation. The paper's main aim is to study the fundamental challenges in using object detection and segmentation of image instances. This paper proposes a novel algorithm for the multi-object image segmentation algorithm in three stages. A novel backbone approach improves the image recognition algorithm by extracting low and high characteristic levels from the given images in the first stage.
This enhances ResNet as the fundamental building block and connects it with the squeeze-and-excitation (SENet) architecture for each ResNet blocks. The Region Proposal Network (RPN) is used to determine the placement of the object item followed by the third stage, which suggests an average position RoI layer to determine the optimal boundaries of the instance segmentation. The experiments are conducted and validated using a standard benchmark images dataset, called COCO. The proposed algorithm's performance is validated using standard evaluation criteria and compared against the recent image segmentation algorithms that use object instances. The results show that the proposed algorithm gets better results than other well-known segmentation algorithms in terms of average accuracy over IoU (AP) thresholds measures using various thresholds.
Key words: Deep learning; Multi-object; Recognition; Instance segmentation; Average position RoI layer.
|
|
|
|